In conclusion, Altris AI has built its platform with a strong commitment to ethical AI principles, ensuring patient data protection, transparency, and compliance with global regulations like GDPR HIPAA, EU AI Act. The system is designed to support, not replace, eye care professionals by enhancing diagnostic accuracy and improving early detection of diseases. By emphasizing machine training ethics, patient-related rights, and the usability of their AI tool, Altris AI fosters trust in healthcare technology while maintaining high standards of transparency, accountability, and human oversight in medical decision-making.
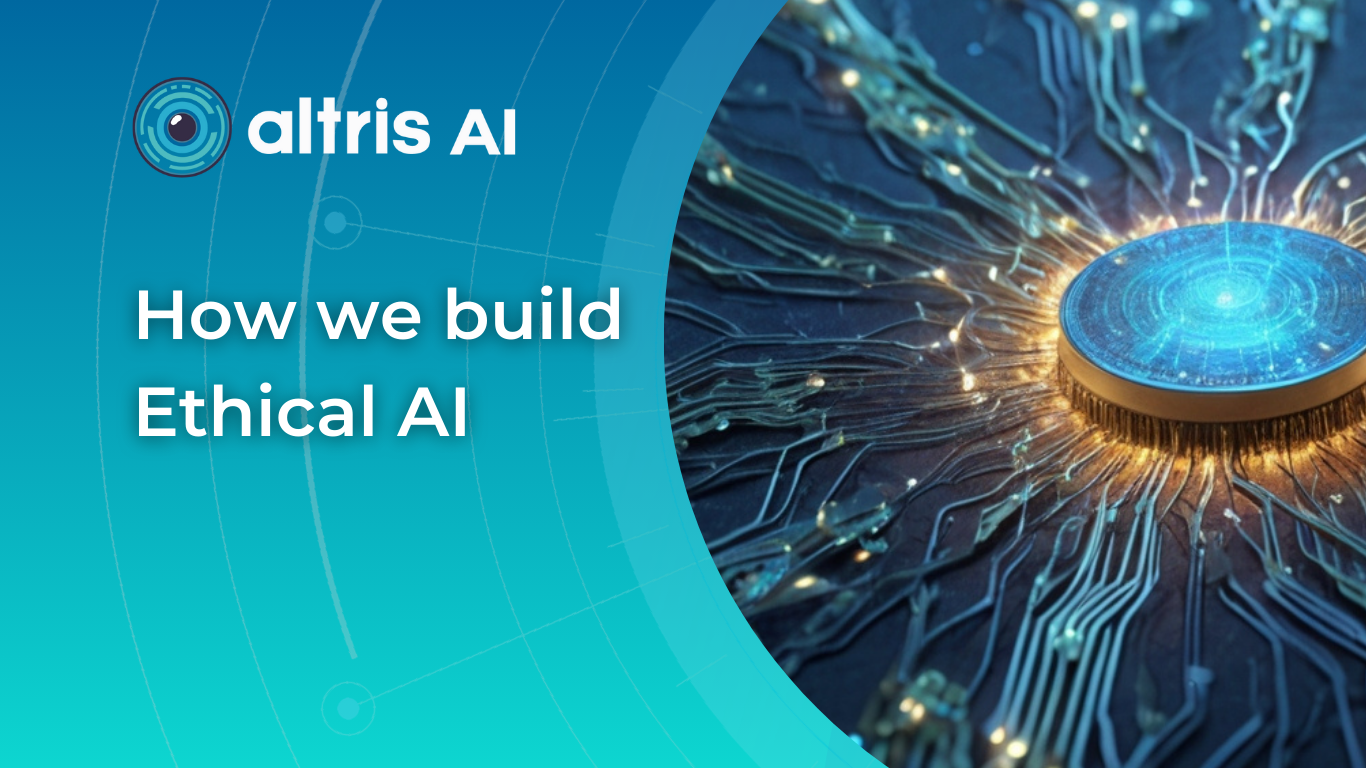
How we build Ethical AI at Altris AI
As the co-owner of the AI HealthTech startup, I get many questions regarding biases and the security of our AI algorithm. After all, Altris AI works directly with patients’ data, which is why these questions are inevitable and even expected. So, I decided to share our approach to building Altris AI as an ethical AI system.
From the very first moments of the company’s creation, I knew that AI and healthcare were two topics that had to be handled very carefully. That is why we ensured that every aspect of the AI platform creation aligned with modern security and ethics guidelines.
It’s like building a house: you need to take care of the foundation before getting to the walls, roof, and decor. Without it, everything will fall sooner or later. Ethical principles of AI are this foundation.
The following aspects of Ethical AI were the most important for us: machine training ethics, machine accuracy ethics, patient-related ethics, eye care specialists-related ethics, usefulness, usability, and efficiency.
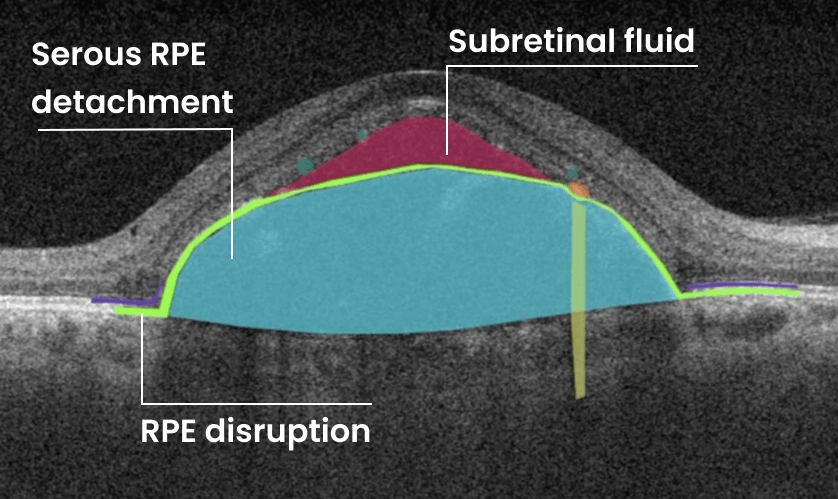
FDA-cleared AI for OCT analysis
Demo Account
Get brochure
1. Machine Training Ethics
To create an accurate algorithm capable of analyzing OCT scans, we needed to train it for years. When it comes to machine training, we speak about data for this training. There are 2 major aspects of machine training ethics that need to be discussed: data ownership and data protection.
Data ownership/Data privacy indicates authority to control, process, or access data. By default, all patients’ data belongs exclusively to patients; no one owns it and sells it to a third party. For Altris AI machine training, all the data was obtained from patients directly who voluntarily agreed to share it and signed relevant documents.
More than that, no client’s data, under any circumstances, is used to train the Altris AI.
Data protection
- GDPR
Currently, there are the following regulations to protect the confidentiality of patients’ data. The European Union (EU) has legislatures of General Data Protection Regulation (GDPR), Cybersecurity Directive, and Medical Devices Regulation.
- HIPAA
In the US, the Health Insurance Portability and Accountability Act (HIPAA) is suggested as a counterpart for European legislation to cover wider confidentiality issues in medical data.
At Altris AI, we obtained EU certification and ensured that all data is GDPR and HIPAA-compliant. This also applies to all the patients’ data we receive.
- European Union Artificial Intelligence Act
Provider obligations
As a provider of a high-risk AI system, we comply with the obligations listed under Article 16.
High-risk obligations
Under Article 6, high-risk obligations apply to systems that are considered a ‘safety component’ of the kind listed in Annex I Section A, and to systems that are considered a ‘High-risk AI system’ under Annex III.
At Altris AI we followed these obligations:
- Established and implemented risk management processes according to Article 9.
- Used high-quality training, validation, and testing data according to Article 10.
- Established documentation and design logging features according to Article 11 and Article 12.
- Ensured an appropriate level of transparency and provided information to users according to Article 13.
- Ensured human oversight measures are built into the system and/or implemented by users according to Article 14.
- Ensured robustness, accuracy, and cybersecurity according to Article 15.
- Set up a quality management system according to Article 17.
Transparency Obligations
At Altris AI we also followed the transparency obligations under Article 50:
- The AI system, the provider or the user must inform any person exposed to the system in a timely, clear manner when interacting with an AI system, unless obvious from context.
- Where appropriate and relevant include information on which functions are AI-enabled, if there is human oversight, who is responsible for decision-making, and what the rights to object and seek redress are.
2. Machine Accuracy Ethics.
Data transparency.
Where transparency in medical AI should be sought?
Transparency in Data Training:
1. What data was the model trained on? Including population characteristics and demographics.
The model’s proprietary training data set was collected from patients from several clinics who consented to share their data anonymously for research purposes. The dataset includes diverse and extensive annotated data from various OCT scanners, encompassing a range of biomarkers and diseases. It does not specifically target or label demographic information, and no population or demographic information was collected.
2. How was the model trained? Including parameterization and tuning performed.
The training process for the deep learning model involves several steps:
- Data Annotation: Medical experts annotated the data, creating the ground truth for biomarker segmentation.
- Data Preprocessing: The data is augmented using unsupervised techniques (e.g., albumentations library) to increase diversity during training.
- Model Architecture: The model’s architecture is based on the UNet model with ResNet backbones, incorporating additional training techniques specifically engineered for OCT images.
- Training Process: The model is trained using supervised learning techniques to predict the output biomarker segmentation mask and diagnosis label, employing backpropagation and gradient descent to minimize the loss function.
- Parameterization: The model has millions of parameters (weights) adjusted during training. Hyperparameters such as learning rate, batch size, and the number of layers are tuned to optimize performance.
- Tuning: Hyperparameter tuning is performed using techniques like grid search, random search, or Bayesian optimization to find the optimal set of parameters that improve the model’s performance on validation data.
3. How has the model been trained to avoid discrimination?
The model training uses a wide variety of data to ensure exposure to different perspectives, reducing the likelihood of reinforcing a single viewpoint. No data related to race, gender identification, or other sensitive attributes is used at any stage of the model’s lifecycle (training, validation, inference). The model solely requires OCT images without additional markers or information.
4. How generalizable is the model? Including what validation has been performed and how do you get comfortable that it generalizes well.
- Validation Methods: The model is validated using a variety of images that were not seen during training.
- Performance Metrics: Metrics like Dice and F1 score are used to evaluate the model’s performance.
- Cross-Domain Testing: The model is tested on images from different OCT scanners and time frames to ensure it can generalize well.
- User Feedback: Real-world usage and feedback help identify areas where the model may not generalize well, allowing for continuous improvement.
5. How explainable is the model? Including what explainability testing has been done, if any.
Explainability Techniques: Techniques like SHAP (SHapley Additive exPlanations), GradCAM, and activation visualization are used to understand which parts of the input images the model focuses on when making predictions.
Medical Expert Testing: Regular testing and analysis are conducted to ensure that the model’s detections make sense to medical experts and that the model’s decisions align with logical and reasonable patterns.
Any AI system is opaque (unintelligible) for two reasons:
- Innate complexity of the system itself.
- Intentional proprietary design for the sake of secrecy and proprietary interests.
Biases. In most instances, an AI tool that gives a wrong decision usually reflects biases inherent in the training data. Biases might be racial, ethnic, genetic, regional, or gender-based.
There should not be any bias related to race and ethnicity because there is no evidence that biomarkers and pathologies manifest themselves differently in patients of different races and ethnicities. Altris AI uses sufficiently diverse gender and age-related data to provide accurate results for OCT analysis.
3. Patient-related ethics.
Patient-related ethics in AI are based on the rights of beneficence, nonmaleficence (safety), autonomy, and justice. Patients exercise their rights either explicitly through informed consent or implicitly through norms of confidentiality or regulatory protections.
Informed Consent.
Informed consent is based on the principle of autonomy. It could authorize the partial or complete role of algorithms in health care services and detail the process of reaching diagnostic or therapeutic decisions by machines. Clinicians should explain the details of these processes to their patients. Patients should have the choice to opt in or out of allowing their data to be handled, processed, and shared.
As these rights can be enabled by eye care professionals, they remain on the side of eye care professionals in our case. However, eye care professionals who use Altris AI not only inform patients about using AI for OCT scan analysis but also use the system to educate patients with the help of color coding.
Confidentiality.
Patients’ confidentiality is a legal obligation and a code of conduct. Confidentiality involves the responsibility of those entrusted to handle and protect patient’s data.
All the data that is used inside the Altris AI platform is anonymized and tokenized, and only eye care professionals who work with patients see any personal information. For the Altris AI team, this data is viewed as a programming code.
4. Eye care specialist-related ethics.
AI systems, like Altris AI, are unable to work 100% autonomously, and therefore, eye care specialists who use them should also make ethical decisions when working with AI.
Overreliance on AI. One of the important aspects of physician-related ethics is overreliance on AI during diagnostic decisions. We never cease to repeat that Altris AI is not a diagnostic tool in any sense; it is a decision-making support tool. The final decision will always be made by an eye care professional. It is an eye care professional who must take into consideration the patient’s clinical history, the results of other diagnostic procedures, lab test results, concomitant diseases, and conclusions from the dialogue with the patient to make the final decision.
Substitution of Doctors’ Role. Considering the information mentioned above, it is important to clarify the aspect of substituting eye care specialists. It should always be kept in mind that the aim of adopting AI is to augment and assist doctors, not to replace them.
Empathy. Empathetic skills and knowledge need to be further incorporated into medical education and training programs. AI performing some tasks offers space for doctors to utilize empathy in medical education and training.
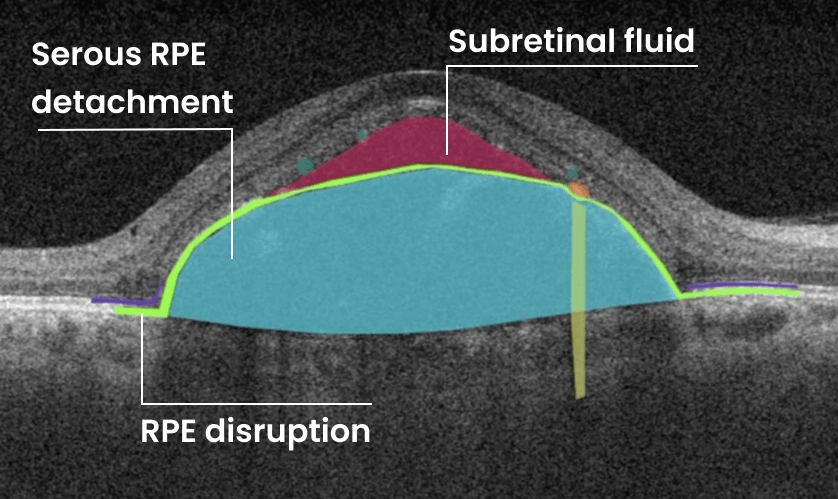
FDA-cleared AI for OCT analysis
Demo Account
Get brochure
5. Usefulness, Usability, and Efficacy.
According to the Coalition for Health AI (CHAI) checklist, AI in healthcare must be, first of all, useful, usable, and efficient.
To be useful, an AI solution must provide a specific benefit to patients and/or healthcare delivery and prove to be not only valid and reliable but also usable and effective. The benefit of an AI solution can be measured based on its effectiveness in achieving intended outcomes and its impact on overall health resulting from both intended and potentially unintended uses. An assessment of benefits should consider the balance between positive effects and adverse effects or risks.
In the case of Altris AI, its usefulness is proved by the clients’ testimonials we receive regularly.
Relatedly, an effective AI solution can be shown to achieve the intended improvement in health compared to existing standards of care, or it can improve existing workflows and processes.
With Altris AI, we make patient screening and triage faster and more effective. We also significantly improve the detection of early pathologies, such as early glaucoma, which are often invisible to the human eye.
Usability presupposes that the AI tool must be easy for healthcare practitioners. Altris AI is actively used by more than 500 eye care businesses worldwide, proving its usability. Moreover, we constantly collect feedback from users and improve the platform’s UI/UX.
Conclusion